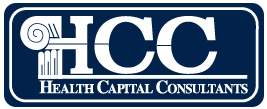
(800)FYI - VALU
Providing Solutions
in the Era of
Healthcare Reform
Founded in 1993, HCC is a nationally recognized healthcare economic fincancial consulting firm
HCC Services
- Valuation Consulting
- Commercial Reasonableness Opinions
- Commercial Payor Reimbursement Benchmarking
- Litigation Support & Expert Witness
- Financial Feasibility Analysis & Modeling
- Intermediary Services
- Certificate of Need
- ACO Value Metrics & Capital Formation
- Strategic Consulting
- Industry Research Services
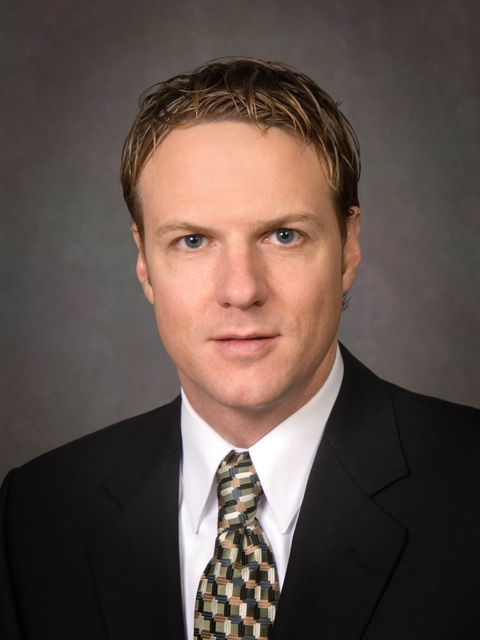
Todd A. Zigrang, MBA, MHA, CVA, ASA, FACHE, ABV, is the President of Health Capital Consultants (HCC), where he focuses on the areas of valuation and financial analysis for hospitals, physician practices, and other healthcare enterprises. Mr. Zigrang has over 30 years of experience providing valuation, financial, transaction and strategic advisory services nationwide in over 2,000 transactions and joint ventures. Mr. Zigrang is also considered an expert in the field of healthcare compensation for physicians, executives and other professionals.
Mr. Zigrang is the co-author of "The Adviser’s Guide to Healthcare – 2nd Edition" [2015 – AICPA], numerous chapters in legal treatises and anthologies, and peer-reviewed and industry articles such as: The Accountant’s Business Manual (AICPA); Valuing Professional Practices and Licenses (Aspen Publishers); Valuation Strategies; Business Appraisal Practice; and, NACVA QuickRead. In addition to his contributions as an author, Mr. Zigrang has served as faculty before professional and trade associations such as the American Society of Appraisers (ASA); the National Association of Certified Valuators and Analysts (NACVA); Physician Hospitals of America (PHA); the Institute of Business Appraisers (IBA); the Healthcare Financial Management Association (HFMA); and, the CPA Leadership Institute.
Mr. Zigrang holds a Master of Science in Health Administration (MHA) and a Master of Business Administration (MBA) from the University of Missouri at Columbia. He is a Fellow of the American College of Healthcare Executives (FACHE) and holds the Certified Valuation Analyst (CVA) designation from NACVA. Mr. Zigrang also holds the Accredited Senior Appraiser (ASA) designation from the American Society of Appraisers, where he has served as President of the St. Louis Chapter. He is also a member of the America Association of Provider Compensation Professionals (AAPCP), AHLA, AICPA, NACVA, NSCHBC, and, the Society of OMS Administrators (SOMSA).
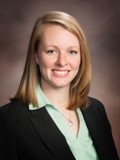
Jessica L. Bailey-Wheaton, Esq., is Senior Vice President and General Counsel of HCC. Her work focuses on the areas of Certificate of Need (CON) preparation and consulting, as well as project management and consulting services related to the impact of both federal and state regulations on healthcare transactions. In that role, Ms. Bailey-Wheaton provides research services necessary to support certified opinions of value related to the Fair Market Value and Commercial Reasonableness of transactions related to healthcare enterprises, assets, and services.
She serves on the editorial board of NACVA’s The Value Examiner and is the current Co-Chair of the American Bar Association (ABA) Health Law Section’s Membership Committee and the Young Lawyer Division (YLD) Liaison to the ABA Health Law Section Governing Council. She has previously presented before the ABA, American Health Law Association (AHLA). NACVA, the National Society of Certified Healthcare Business Consultants (NSCHBC), and the American College of Surgeons.
Ms. Bailey-Wheaton is a member of the Missouri and Illinois Bars and holds a J.D., with a concentration in Health Law, from Saint Louis University School of Law.
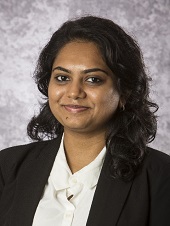
Janvi R. Shah, MBA, MSF, CVA, serves as Senior Financial Analyst of HCC. Mrs. Shah holds a M.S. in Finance from Washington University Saint Louis and the CVA designation from NACVA. She develops fair market value and commercial reasonableness opinions related to healthcare enterprises, assets, and services. In addition she prepares, reviews and analyzes forecasted and pro forma financial statements to determine the most probable future net economic benefit related to healthcare enterprises, assets, and services and applies utilization demand and reimbursement trends to project professional medical revenue streams and ancillary services and technical component (ASTC) revenue streams.
HCC's Leadership and Capabilities